Qwen swings for a double with 2.5-Omni-3B model that runs on consumer PCs, laptops
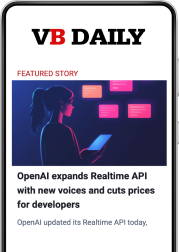
Join our daily and weekly newsletters for the latest updates and exclusive content on industry-leading AI coverage. Learn More
Chinese e-commerce and cloud giant Alibaba isn’t taking the pressure off other AI model providers in the U.S. and abroad.
Just days after releasing its new, state-of-the-art open source Qwen3 large reasoning model family, Alibaba’s Qwen team today released Qwen2.5-Omni-3B, a lightweight version of its preceding multimodal model architecture designed to run on consumer-grade hardware without sacrificing broad functionality across text, audio, image, and video inputs.
Qwen2.5-Omni-3B is a scaled-down, 3-billion-parameter variant of the team’s flagship 7 billion parameter (7B) model. (Recall parameters refer to the number of settings governing the model’s behavior and functionality, with more typically denoting more powerful and complex models).
While smaller in size, the 3B version retains over 90% of the larger model’s multimodal performance and delivers real-time generation in both text and natural-sounding speech.
A major improvement comes in GPU memory efficiency. The team reports that Qwen2.5-Omni-3B reduces VRAM usage by over 50% when processing long-context inputs of 25,000 tokens. With optimized settings, memory consumption drops from 60.2 GB (7B model) to just 28.2 GB (3B model), enabling deployment on 24GB GPUs commonly found in high-end desktops and laptop computers — instead of the larger dedicated GPU clusters or workstations found in enterprises.
According to the developers, it achieves this through architectural features such as the Thinker-Talker design and a custom position embedding method, TMRoPE, which aligns video and audio inputs for synchronized comprehension.
However, the licensing terms specify for research only — meaning enterprises cannot use the model to build commercial products unless they obtain a separate license from Alibaba’s Qwen Team, first.
The announcement follows increasing demand for more deployable multimodal models and is accompanied by performance benchmarks showing competitive results relative to larger models in the same series.
The model is now freely available for download from:
Developers can integrate the model into their pipelines using Hugging Face Transformers, Docker containers, or Alibaba’s vLLM implementation. Optional optimizations such as FlashAttention 2 and BF16 precision are supported for enhanced speed and reduced memory consumption.
Benchmark performance shows strong results even approaching much larger parameter models
Despite its reduced size, Qwen2.5-Omni-3B performs competitively across key benchmarks:
The narrow performance gap in video and speech tasks highlights the efficiency of the 3B model’s design, particularly in areas where real-time interaction and output quality matter most.
Real-time speech, voice customization, and more
Qwen2.5-Omni-3B supports simultaneous input across modalities and can generate both text and audio responses in real time.
The model includes voice customization features, allowing users to choose between two built-in voices—Chelsie (female) and Ethan (male)—to suit different applications or audiences.
Users can configure whether to return audio or text-only responses, and memory usage can be further reduced by disabling audio generation when not needed.
Community and ecosystem growth
The Qwen team emphasizes the open-source nature of its work, providing toolkits, pretrained checkpoints, API access, and deployment guides to help developers get started quickly.
The release also follows recent momentum for the Qwen2.5-Omni series, which has reached top rankings on Hugging Face’s trending model list.
Junyang Lin from the Qwen team commented on the motivation behind the release on X, stating, “While a lot of users hope for smaller Omni model for deployment we then build this.”
What it means for enterprise technical decision-makers
For enterprise decision makers responsible for AI development, orchestration, and infrastructure strategy, the release of Qwen2.5-Omni-3B may appear, at first glance, like a practical leap forward. A compact, multimodal model that performs competitively against its 7B sibling while running on 24GB consumer GPUs offers real promise in terms of operational feasibility. But as with any open-source technology, licensing matters—and in this case, the license draws a firm boundary between exploration and deployment.
The Qwen2.5-Omni-3B model is licensed for non-commercial use only under Alibaba Cloud’s Qwen Research License Agreement. That means organizations can evaluate the model, benchmark it, or fine-tune it for internal research purposes—but cannot deploy it in commercial settings, such as customer-facing applications or monetized services, without first securing a separate commercial license from Alibaba Cloud.
For professionals overseeing AI model lifecycles—whether deploying across customer environments, orchestrating at scale, or integrating multimodal tools into existing pipelines—this restriction introduces important considerations. It may shift Qwen2.5-Omni-3B’s role from a deployment-ready solution to a testbed for feasibility, a way to prototype or evaluate multimodal interactions before deciding whether to license commercially or pursue an alternative.
Those in orchestration and ops roles may still find value in piloting the model for internal use cases—like refining pipelines, building tooling, or preparing benchmarks—so long as it remains within research bounds. Data engineers or security leaders might likewise explore the model for internal validation or QA tasks, but should tread carefully when considering its use with proprietary or customer data in production environments.
The real takeaway here may be about access and constraint: Qwen2.5-Omni-3B lowers the technical and hardware barrier to experimenting with multimodal AI, but its current license enforces a commercial boundary. In doing so, it offers enterprise teams a high-performance model for testing ideas, evaluating architectures, or informing make-vs-buy decisions—yet reserves production use for those willing to engage Alibaba for a licensing discussion.
In this context, Qwen2.5-Omni-3B becomes less a plug-and-play deployment option and more a strategic evaluation tool—a way to get closer to multimodal AI with fewer resources, but not yet a turnkey solution for production.