Enhancing Conversational AI: Strategies to Reduce Latency
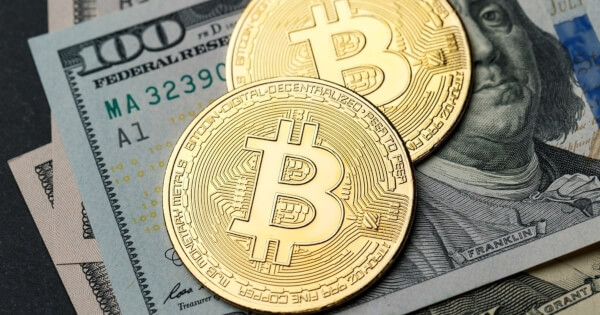
Zach Anderson
Jan 24, 2025 13:27
Latency optimization is crucial for conversational AI’s success. Discover strategies to minimize delays and enhance user experience in AI-driven interactions.
In the realm of conversational AI, minimizing latency is paramount to delivering a seamless and human-like interaction experience. The ability to converse without noticeable delays is what distinguishes superior applications from merely functional ones, according to ElevenLabs.
Understanding Latency in Conversational AI
Conversational AI aims to emulate human dialogue by ensuring fluid communication, which involves complex processes that can introduce latency. Each step, from converting speech to text to generating responses, contributes to the overall delay. Thus, optimizing these processes is vital to enhance the user experience.
The Four Core Components of Conversational AI
Conversational AI systems typically involve four main components: speech-to-text, turn-taking, text processing via large language models (LLMs), and text-to-speech. These components, although executed in parallel, each add to the latency. Unlike other systems where a single bottleneck might dominate, conversational AI’s latency is a cumulative effect of these processes.
Component Analysis
Automatic Speech Recognition (ASR): Often termed as speech-to-text, ASR converts spoken words into text. The latency here is not in text generation but in the time taken from speech end to text completion.
Turn-Taking: Efficiently managing dialogue turns between the AI and user is crucial to prevent awkward pauses.
Text Processing: Utilizing LLMs to process text and generate meaningful responses quickly is essential.
Text-to-Speech: Finally, converting the generated text back into speech with minimal delay completes the interaction.
Strategies for Latency Optimization
Various techniques can be employed to optimize latency in conversational AI. Leveraging advanced algorithms and processing techniques can significantly reduce delays. Streamlining the integration of these components ensures faster processing times and a more natural conversation flow.
Furthermore, advancements in hardware and cloud computing have enabled more efficient processing and faster response times, allowing developers to push the boundaries of what conversational AI can achieve.
Future Prospects
As technology continues to evolve, the potential for further reducing latency in conversational AI is promising. Ongoing research and development in AI and machine learning are expected to yield more sophisticated solutions, enhancing the realism and efficiency of AI-driven interactions.
Image source: Shutterstock