H2O.ai improves AI agent accuracy with predictive models
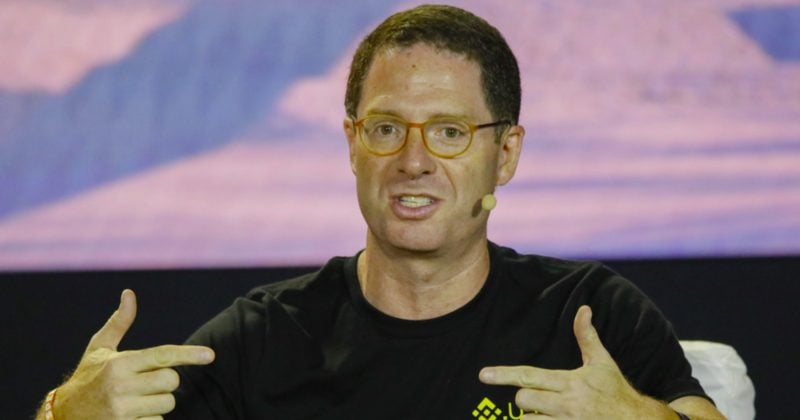
Join our daily and weekly newsletters for the latest updates and exclusive content on industry-leading AI coverage. Learn More
Open-source AI platform provider H2O.ai believes a blend of generative and predictive AI models makes for more consistent responses which enterprises want from an AI agent.
H2O.ai launched its new multi-agent platform that blends generative and predictive AI and is now generally available.
The platform, h2oGPTe, uses the company’s AI models Mississippi and Danube, but can also access other large and small language models available. The company said h2oGPTe works in air-gapped, on-premise and cloud systems.
Sri Ambati, founder and CEO of H2O, told VentureBeat that having both generative and predictive AI gives enterprises more confidence that the agents will work exactly as they need without compromising security.
“The number one problem with agents is consistency. Can I get a consistent response from an [large language model] LLM for the same prompt? I think you get two different, like multiple responses right now,” Ambati said. “But you can bring multiple models that negotiate, plan and deliver an outcome. Think of it as humans can have a bit of variability with each other, but you still expect a consistent response, and that’s the domain of predictive AI combined with generative AI.”
Ambati explained that generative AI models are “decent at content generation and very good at code generation,” but predictive models bring more scenario simulation to the table. He said the predictive models bring consistency to agentic responses because these do not just generate responses but learn from patterns in data.
The platform is built for finance, telecommunications, healthcare and government enterprises that need to manage multi-step tasks. H2O.ai’s agent works best for organizations that want to get insights into their business and not just a guide that runs through their workflows. This is because agents within the h2oGPTe platform can read multimodal data like charts and craft answers to questions like “Should my company sell more dolls this year?” that consider the enterprise’s historical financial data or market trend information they store.
Multimodal agents
Like other AI agents, h2oGPTe automates workflow tasks so human employees don’t have to do those activities themselves. Ambati said the multimodal capabilities of H2O.ai’s agents open up more information that it can learn from to offer the best, most consistent answers to users.
The company said the agents can also create PDF documents with charts and tables grounded in enterprise data to visualize information for the human user. H2O.ai ensured that the agents cite their sources for data traceability and offer customizable guardrails.
H2O.ai’s agentic platform builds in model testing, including automated question generation, where an AI model will create variations of a prompt and barrage the agent with questions to see if it consistently responds. It also has a dashboard where people can identify which type of database, model, or part of the workflow the agents tapped.
Consistency and accuracy in agents
With the hype around AI agents predicted to continue to the following year, there is a need to ensure agents provide value to enterprises, including performing consistently, reliably and accurately.
Reliability is critical because AI agents are meant to automate a large portion of an enterprise’s workflow without human intervention.
H2O.ai’s approach of blending generative and predictive models is one way, but other companies are also looking at ways to ensure AI agents don’t cause trouble for enterprises. The startup xpander.ai introduced its Agent Graph System for multi-step agents. Salesforce also released to a limited preview its Agentforce Testing Center to test agent response consistency.