Medical training’s AI leap: How agentic RAG, open-weight LLMs and real-time case insights are shaping a new generation of doctors at NYU Langone
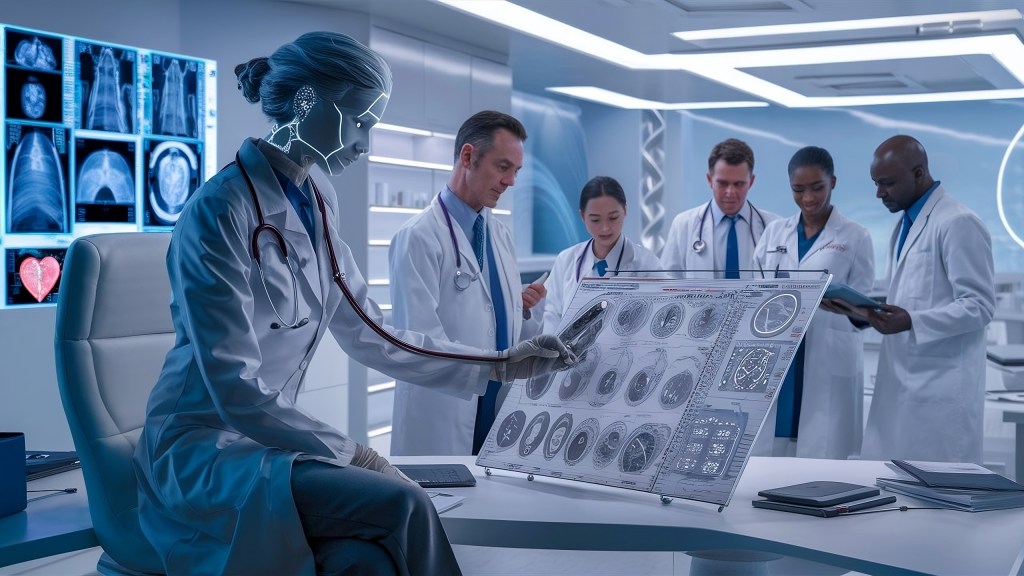
Join our daily and weekly newsletters for the latest updates and exclusive content on industry-leading AI coverage. Learn More
Patient data records can be convoluted and sometimes incomplete, meaning doctors don’t always have all the information they need readily available. Added to this is the fact that medical professionals can’t possibly keep up with the barrage of case studies, research papers, trials and other cutting-edge developments coming out of the industry.
New York City-based NYU Langone Health has come up with a novel approach to tackle these challenges for the next generation of doctors.
The academic medical center — which comprises NYU Grossman School of Medicine and NYU Grossman Long Island School of Medicine, as well as six inpatient hospitals and 375 outpatient locations — has developed a large language model (LLM) that serves as a respected research companion and medical advisor.
Every night, the model processes electronic health records (EHR), matching them with relevant research, diagnosis tips and essential background information that it then delivers in concise, tailored emails to residents the following morning. This is an elemental part of NYU Langone’s pioneering approach to medical schooling — what it calls “precision medical education” that uses AI and data to provide highly customized student journeys.
“This concept of ‘precision in everything’ is needed in healthcare,” Marc Triola, associate dean for educational informatics and director of the Institute for Innovations in Medical Education at NYU Langone Health, told VentureBeat. “Clearly the evidence is emerging that AI can overcome many of the cognitive biases, errors, waste and inefficiencies in the healthcare system, that it can improve diagnostic decision-making.”
How NYU Langone is using Llama to enhance patient care
NYU Langone is using an open-weight model built on the latest version of Llama-3.1-8B-instruct and the open-source Chroma vector database for retrieval-augmented generation (RAG). But it’s not just accessing documents — the model is going beyond RAG, actively employing search and other tools to discover the latest research documents.
Each night, the model connects to the facility’s EHR database and pulls out medical data for patients seen at Langone the previous day. It then searches for basic background information on diagnoses and medical conditions. Using a Python API, the model also performs a search of related medical literature in PubMed, which has “millions and millions of papers,” Triola explained. The LLM sifts through reviews, deep-dive papers and clinical trials, selecting a couple of the seemingly most relevant and “puts it all together in a nice email.”
Early the following morning, medical students and internal medicine, neurosurgery and radiation oncology residents receive a personalized email with detailed patient summaries. For instance, if a patient with congestive heart failure had been in for a checkup the previous day, the email will provide a refresher on the basic pathophysiology of heart conditions and information about the latest treatments. It also offers self-study questions and AI-curated medical literature. Further, it may give pointers about steps the residents could take next or actions or details they may have overlooked.
“We’ve gotten great feedback from students, from residents and from the faculty about how this is frictionlessly keeping them up to date, how they’re incorporating this in the way they make choices about a patient’s plan of care,” said Triola.
A key success metric for him personally was when a system outage halted the emails for a few days — and faculty members and students complained they weren’t receiving the morning nudges they had come to rely on.
“Because we’re sending these emails right before our doctors start rounds — which is among the craziest and busiest times of the day for them — and for them to notice that they weren’t getting these emails and miss them as a part of their thinking was awesome,” he said.
Transforming the industry with precision medical education
This sophisticated AI retrieval system is fundamental to NYU Langone’s precision medical education model, which Triola explained is based on “higher density, frictionless” digital data, AI and strong algorithms.
The institution has collected vast amounts of data over the past decade about students — their performance, the environments they’re taking care of patients in, the EHR notes they’re writing, the clinical decisions they’re making and the way they reason through patient interactions and care. Further, NYU Langone has a vast catalog of all the resources available to medical students, whether those be videos, self-study or exam questions, or online learning modules.
The success of the project is also thanks to the medical facility’s streamlined architecture: It boasts centralized IT, a single data warehouse on the healthcare side and a single data warehouse for education, allowing Langone to marry its various data resources.
Chief medical information officer Paul Testa noted that great AI/ML systems aren’t possible without great data, but “it’s not the easiest thing to do if you’re sitting on unwarehoused data in silos across your system.” The medical system may be large, but it operates as “one patient, one record, one standard.”
Gen AI allowing NYU Langone to move away from ‘one-size-fits-all’ education
As Triola put it, the main question his team has been looking to address is: “How do they link the diagnosis, the context of the individual student and all of these learning materials?”
“All of a sudden we’ve got this great key to unlock that: generative AI,” he said.
This has enabled the school to move away from a “one-size-fits-all” model that has been the norm, whether students intended to become, for example, a neurosurgeon or a psychiatrist — vastly different disciplines that require unique approaches.
It’s important that students get tailored education throughout their schooling, as well as “educational nudges” that adapt to their needs, he said. But you can’t just tell faculty to “spend more time with each individual student” — that’s humanly impossible.
“Our students have been hungry for this, because they recognize that this is a high-velocity period of change in medicine and generative AI,” said Triola. “It absolutely will change…what it means to be a physician.”
Serving as a model for other medical institutions
Not that there haven’t been challenges along the way. Notably, technical teams have been working through model “immaturity.”
As Triola noted: “It’s fascinating how expansive and accurate their embedded knowledge is, and sometimes how limited. It’ll work perfectly, predictably, 99 times in a row, and then on the 100th time it’ll make an interesting set of choices.”
For instance, early on in development, the LLMs couldn’t differentiate between an ulcer on the skin and an ulcer in the stomach, which are “not related conceptually at all,” Triola explained. His team has since focused on prompt refining and grounding, and the result has been “remarkable.”
In fact, his team is so confident in the stack and process that they believe it can serve as a great example for others to follow. “We were favoring open source and open weight because we wanted to get to the point where we could say, ‘Hey, other medical schools, many of whom don’t have a lot of resources, you can do this on the cheap,’” Triola explained.
Testa agreed: “Is it reproducible? Is it something we want to disseminate? Absolutely, we want to disseminate it across healthcare.”
Reassessing ‘sacrosanct’ practices in medicine
Understandably, there’s much concern across the indusry about nuanced biases that might be baked into AI systems. However, Triola pointed out that that’s not a huge concern in this use case, as it’s a relatively straightforward task for AI. “It’s searching, it’s choosing from a list, it’s summarizing,” he noted.
Rather, one of the biggest surfaced concerns is around unskilling or deskilling. Here’s a correlation: Those of a certain vintage might remember learning cursive in elementary school — yet they likely have forgotten the skill because they’ve found rare occasion to use it in their adult life. Now, it’s near obsolete, rarely taught in today’s primary education.
Triola pointed out that there are “sacrosanct” parts of being a physician, and that some are resistant to give those up to AI or digital systems “in any way, shape or form.” For example, there’s a perception that young doctors should be actively researching and nose-down in the latest literature whenever they’re not in a clinical setting. But the amount of medical knowledge available today and the “frenetic pace” of clinical medicine demands a different way of doing things, Triola emphasized.
When it comes to researching and retrieving information, he noted: “AI does it better, and that’s an uncomfortable truth that many people are hesitant to believe.”
Instead, he posited: “Let’s say that this is going to give superpowers to doctors and figure out the co-pilot relationship between the human and AI, not the competitive relationship of who’s going to do what.”