Purpose-built AI hardware: Smart strategies for scaling infrastructure
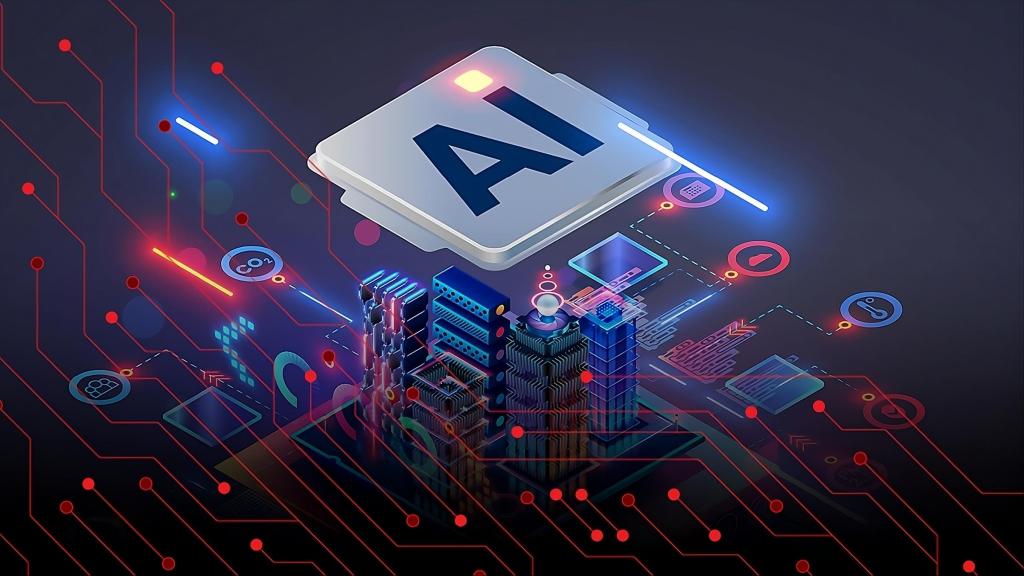
This article is part of VentureBeat’s special issue, “AI at Scale: From Vision to Viability.” Read more from this special issue here.
This article is part of VentureBeat’s special issue, “AI at Scale: From Vision to Viability.” Read more from the issue here.
Enterprises can look forward to new capabilities — and strategic decisions — around the crucial task of creating a solid foundation for AI expansion in 2025. New chips, accelerators, co-processors, servers and other networking and storage hardware specially designed for AI promise to ease current shortages and deliver higher performance, expand service variety and availability, and speed time to value.
The evolving landscape of new purpose-built hardware is expected to fuel continued double-digit growth in AI infrastructure that IDC says has lasted 18 straight months. The IT firm reports that organizational buying of compute hardware (primarily servers with accelerators) and storage hardware infrastructure for AI grew 37% year over-year in the first half of 2024. Sales are forecast to triple to $100 billion a year by 2028.
“Combined spending on dedicated and public cloud infrastructure for AI is expected to represent 42% of new AI spending worldwide through 2025” writes Mary Johnston Turner, research VP for digital infrastructure strategies at IDC.
The main highway for AI expansion
Many analysts and experts say these staggering numbers illustrate that infrastructure is the main highway for AI growth and enterprise digital transformation. Accordingly, they advise, technology and business leaders in mainstream companies should make AI infrastructure a crucial strategic, tactical and budget priority in 2025.
“Success with generative AI hinges on smart investment and robust infrastructure,”
said Anay Nawathe, director of cloud and infrastructure delivery at ISG, a global research and advisory firm. “Organizations that benefit from generative AI redistribute their
budgets to focus on these initiatives.”
As evidence, Nawathe cited a recent ISG global survey that found that proportionally, organizations had ten projects in the pilot phase and 16 in limited deployment, but only six deployed at scale. A major culprit, says Nawathe, was the current infrastructure’s inability to affordably, securely, and performantly scale.” His advice? “Develop comprehensive purchasing practices and maximize GPU availability and utilization, including investigating specialized GPU and AI cloud services.”
Others agree that when expanding AI pilots, proof of concepts or initial projects, it’s essential to choose deployment strategies that offer the right mix of scalability, performance, price, security and manageability.
Experienced advice on AI infrastructure strategy
To help enterprises build their infrastructure strategy for AI expansion, VentureBeat consulted more than a dozen CTOs, integrators, consultants and other experienced industry experts, as well as an equal number of recent surveys and reports.
The insights and advice, along with hand-picked resources for deeper exploration, can help guide organizations along the smartest path for leveraging new AI hardware and help drive operational and competitive advantages.
Smart strategy 1: Start with cloud services and hybrid
For most enterprises, including those scaling large language models (LLMs), experts say the best way to benefit from new AI-specific chips and hardware is indirectly — that is,
through cloud providers and services.
That’s because much of the new AI-ready hardware is costly and aimed at giant data centers. Most new products will be snapped up by hyperscalers Microsoft, AWS, Meta and Google; cloud providers like Oracle and IBM; AI giants such as XAI and OpenAI and other dedicated AI firms; and major colocation companies like Equinix. All are racing to expand their data centers and services to gain competitive advantage and keep up with surging demand.
As with cloud in general, consuming AI infrastructure as a service brings several advantages, notably faster jump-starts and scalability, freedom from staffing worries and the convenience of pay-go and operational expenses (OpEx) budgeting. But plans are still emerging, and analysts say 2025 will bring a parade of new cloud services based on powerful AI optimized hardware, including new end-to-end and industry-specific options.
Smart strategy 2: DIY for the deep-pocketed and mature
New optimized hardware won’t change the current reality: Do it yourself (DIY) infrastructure for AI is best suited for deep-pocketed enterprises in financial services, pharmaceuticals, healthcare, automotive and other highly competitive and regulated industries.
As with general-purpose IT infrastructure, success requires the ability to handle high capital expenses (CAPEX), sophisticated AI operations, staffing and partners with specialty skills, take hits to productivity and take advantage of market opportunities during building. Most firms tackling their own infrastructure do so for proprietary applications with high return on investment (ROI).
Duncan Grazier, CTO of BuildOps, a cloud-based platform for building contractors, offered a simple guideline. “If your enterprise operates within a stable problem space with well-known mechanics driving results, the decision remains straightforward: Does the capital outlay outweigh the cost and timeline for a hyperscaler to build a solution tailored to your problem? If deploying new hardware can reduce your overall operational expenses by 20-30%, the math often supports the upfront investment over a three-year period.”
Despite its demanding requirements, DIY is expected to grow in popularity. Hardware vendors will release new, customizable AI-specific products, prompting more and more mature organizations to deploy purpose-built, finely tuned, proprietary AI in private clouds or on premise. Many will be motivated by faster performance of specific workloads, derisking model drift, greater data protection and control and better cost management.
Ultimately, the smartest near-term strategy for most enterprises navigating the new infrastructure paradigm will mirror current cloud approaches: An open, “fit-for- purpose” hybrid that combines private and public clouds with on-premise and edge.
Smart strategy 3: Investigate new enterprise-friendly AI devices
Not every organization can get their hands on $70,000 high end GPUs or afford $2 million AI servers. Take heart: New AI hardware with more realistic pricing for everyday organizations is starting to emerge .
The Dell AI Factory, for example, includes AI Accelerators, high-performance servers, storage, networking and open-source software in a single integrated package. The company also has announced new PowerEdge servers and an Integrated Rack 5000 series offering air and liquid-cooled, energy-efficient AI infrastructure. Major PC makers continue to introduce powerful new AI-ready models for decentralized, mobile and edge processing.
Veteran industry analyst and consultant Jack E. Gold — president and principal analyst of J. Gold Associates — said he sees a growing role for less expensive options in accelerating adoption and growth of enterprise AI. Gartner projects that by the end of 2026, all new enterprise PCs will be AI-ready.
Smart strategy 4: Double down on basics
The technology might be new. But good news: Many rules remain the same.
“Purpose-built hardware tailored for AI, like Nvidia’s industry-leading GPUs, Google’s TPUs, Cerebras wafer-scale chips and others are making build versus buy decisions much more nuanced,” said ISG’s Nawathe. But he and others point out that the core principles for making these decisions remain largely consistent and familiar. “Enterprises are still evaluating business need, skills availability, cost, usability, supportability and best of breed versus best in class.”
Experienced hands stress that the smartest decisions about whether and how to adopt AI-ready hardware for maximum benefit requires fresh-eyed, disciplined analysis of procurement fundamentals. Specifically: Impact on the larger AI stack of software, data and platforms and a thorough review of specific AI goals, budgets, total cost of ownership (TCO) and ROI, security and compliance requirements, available expertise and compatibility with existing technology.
Energy for operating and cooling are a big X-factor. While much public attention focuses on new, mini nuclear plants to handle AI’s voracious hunger for electricity, analysts say non-provider enterprises must begin factoring in their own energy expenses and the impact of AI infrastructure and usage on their corporate sustainability goals.
Start with use cases, not hardware and technology
In many organizations, the era of AI “science experiments” and “shiny objects” is ending or over. From now on, most projects will require clear, attainable key performance indicators (KPIs) and ROI. This means enterprises must clearly identify the “why” of business value before considering the “how “of technology infrastructure.
“You’d be surprised at how often this basic gets ignored,” said Gold.
No doubt, choosing the best qualitative and quantitative metrics for AI infrastructure and initiatives is a complex, emerging, personalized process.
Get your data house in order first
Likewise, industry experts — not just sellers of data products — stress the importance of a related best practice: Beginning with data. Deploying high-performance (or any) AI infrastructure without ensuring data quality, quantity, availability and other basics will quickly and expensively lead to bad results.
Juan Orlandini, CTO of North America for global solutions and systems integrator Insight Enterprises pointed out: “Buying one of these super highly accelerated AI devices without actually having done the necessary hard work to understand your data, how to use it or leverage it and whether it’s good is like buying a firewall but not understanding how to protect yourself.”
Unless you’re eager to see what garage in/ garbage out (GIGO) on steroids looks like, don’t make this mistake.
And, make sure to keep an eye on the big picture, advises Kjell Carlsson, head of AI strategy at Domino Data Lab, and a former Forrester analyst. He warned: “Enterprises will see little benefit from these new AI hardware offerings without dramatically upgrading their software capabilities to orchestrate, provision and govern this infrastructure across all of the activities of the AI lifecycle.”
Be realistic about AI infrastructure needs
If your company is mostly using or expanding CoPilot, Open AI and other LLMs for productivity, you probably don’t need any new infrastructure for now, said Matthew
Chang, principal and founder of Chang Robotics.
Many large brands, including Fortune 500 manufacturer clients of his Jacksonville, Fl., engineering company, are getting great results using AI-as-a-service. “They don’t have
the computational demands,” he explained, “so, it doesn’t make sense to spend millions of dollars on a compute cluster when you can get the highest-end product in the market, Chat GPT Pro, for $200 a month.”
IDC advises thinking about AI impact on infrastructure and hardware requirements as a spectrum. From highest to lowest impact: Building highly tailored custom models, adjusting pre-trained models with first-party data, contextualizing off the-shelf applications, consuming AI- infused applications “as-is”. How do you determine minimum infrastructure viability for your enterprise? Learn more here.
Stay flexible and open for a fast-changing future
Sales of specialized AI hardware are expected to keep rising in 2025 and beyond. Gartner forecasts a 33% increase, to $92 billion, for AI-specific chip sales in 2025.
On the service side, the growing ranks of GPU cloud providers continue to attract new money, players including Foundry and enterprise customers. An S&P/Weka survey found that more than 30% of enterprises have already used alternate providers for inference and training, often because they couldn’t source GPUs. An oversubscribed $700-million private funding round for Nebius Group, a provider of cloud-based, full-stack AI infrastructure, suggests even wider growth in that sphere.
AI is already moving from training in giant data centers to inference at the edge on AI-enabled smart phones, PCs and other devices. This shift will yield new specialized processors, noted Yvette Kanouff, partner at JC2 Ventures and former head of Cisco’s service provider business. “I’m particularly interested to see where inference chips go in terms of enabling more edge AI, including individual CPE inference-saving resources and latency in run time,” she said.
Because the technology and usage are evolving quickly, many experts caution against getting locked into any service provider or technology. There’s wide agreement that multi-tenancy environments which spread AI infrastructure, data and services across two or more cloud providers — is a sensible strategy for enterprises.
Srujan Akula, CEO and co-founder of The Modern Data Company, goes a step further. Hyperscalers offer convenient end-to-end solutions, he said, but their integrated approaches make customers dependent on a single company’s pace of innovation and capabilities. A better strategy, he suggested , is to follow open standards and decouple storage from compute. Doing so lets an organization rapidly adopt new models and technologies as they emerge, rather than waiting for the vendor to catch up.
“Organizations need the freedom to experiment without architectural constraints,” agreed BuildOps CTO Grazier. “Being locked into an iPhone 4 while the iPhone 16 Pro is available would doom a consumer application, so why should it be any different in this context? The ability to transition seamlessly from one solution to another without the need to rebuild your infrastructure is crucial for maintaining agility and staying ahead in a rapidly evolving landscape.”