Salesforce launches Agentforce Testing Center to put agents through paces
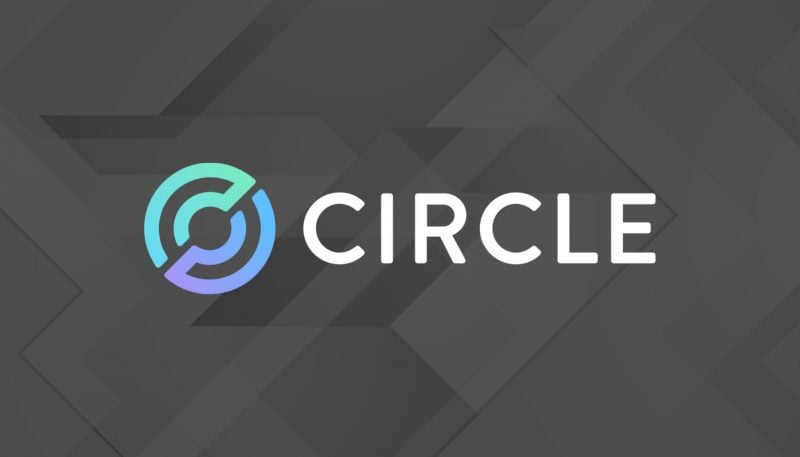
Join our daily and weekly newsletters for the latest updates and exclusive content on industry-leading AI coverage. Learn More
The next phase of agentic AI may just be evaluation and monitoring, as enterprises want to make the agents they’re beginning to deploy more observable.
While AI agent benchmarks can be misleading, there’s a lot of value in seeing if the agent is working the way they want to. To this end, companies are beginning to offer platforms where customers can sandbox AI agents or evaluate their performance.
Salesforce released its agent evaluation platform, Agentforce Testing Center, in a limited pilot Wednesday. General availability is expected in December. Testing Center lets enterprises observe and prototype AI agents to ensure they access the workflows and data they need.
Testing Center’s new capabilities include AI-generated tests for Agentforce, Sandboxes for Agentforce and Data Cloud and monitoring and observability for Agentforce.
AI-generated tests allow companies to use AI models to generate “hundreds of synthetic interactions” to test if agents end up in how often they answer the way companies want. As the name suggests, sandboxes offer an isolated environment to test agents while mirroring a company’s data to reflect better how the agent will work for them. Monitoring and observability let enterprises bring an audit trail to the sandbox when the agents go into production.
Patrick Stokes, executive vice president of product and industries marketing at Salesforce, told VentureBeat that the Testing Center is part of a new class of agents the company calls Agent Lifecycle Management.
“We are positioning what we think will be a big new subcategory of agents,” Stokes said. “When we say lifecycle, we mean the whole thing from genesis to development all the way through deployment, and then iterations of your deployment as you go forward.”
Stokes said that right now, the Testing Center doesn’t have workflow-specific insights where developers can see the specific choices in API, data or model the agents used. However, Salesforce collects that kind of data on its Einstein Trust Layer.
“What we’re doing is building developer tools to expose that metadata to our customers so that they can actually use it to better build their agents,” Stokes said.
Salesforce is hanging its hat on AI agents, focusing a lot of its energy on its agentic offering Agentforce. Salesforce customers can use preset agents or build customized agents on Agentforce to connect to their instances.
Evaluating agents
AI agents touch many points in an organization, and since good agentic ecosystems aim to automate a big chunk of workflows, making sure they work well becomes essential.
If an agent decides to tap the wrong API, it could spell disaster for a business. AI agents are stochastic in nature, like the models that power them, and consider each potential probability before coming up with an outcome. Stokes said Salesforce tests agents by barraging the agent with versions of the same utterances or questions. Its responses are scored as pass or fail, allowing the agent to learn and evolve within a safe environment that human developers can control.
Platforms that help enterprises evaluate AI agents are fast becoming a new type of product offering. In June, customer experience AI company Sierra launched an AI agent benchmark called TAU-bench to look at the performance of conversational agents. Automation company UiPath released its Agent Builder platform in October which also offered a means to evaluate agent performance before full deployment.
Testing AI applications is nothing new. Other than benchmarking model performances, many AI model repositories like AWS Bedrock and Microsoft Azure already let customers test out foundation models in a controlled environment to see which one works best for their use cases.