Transforming real-time monitoring with AI-enhanced digital twins
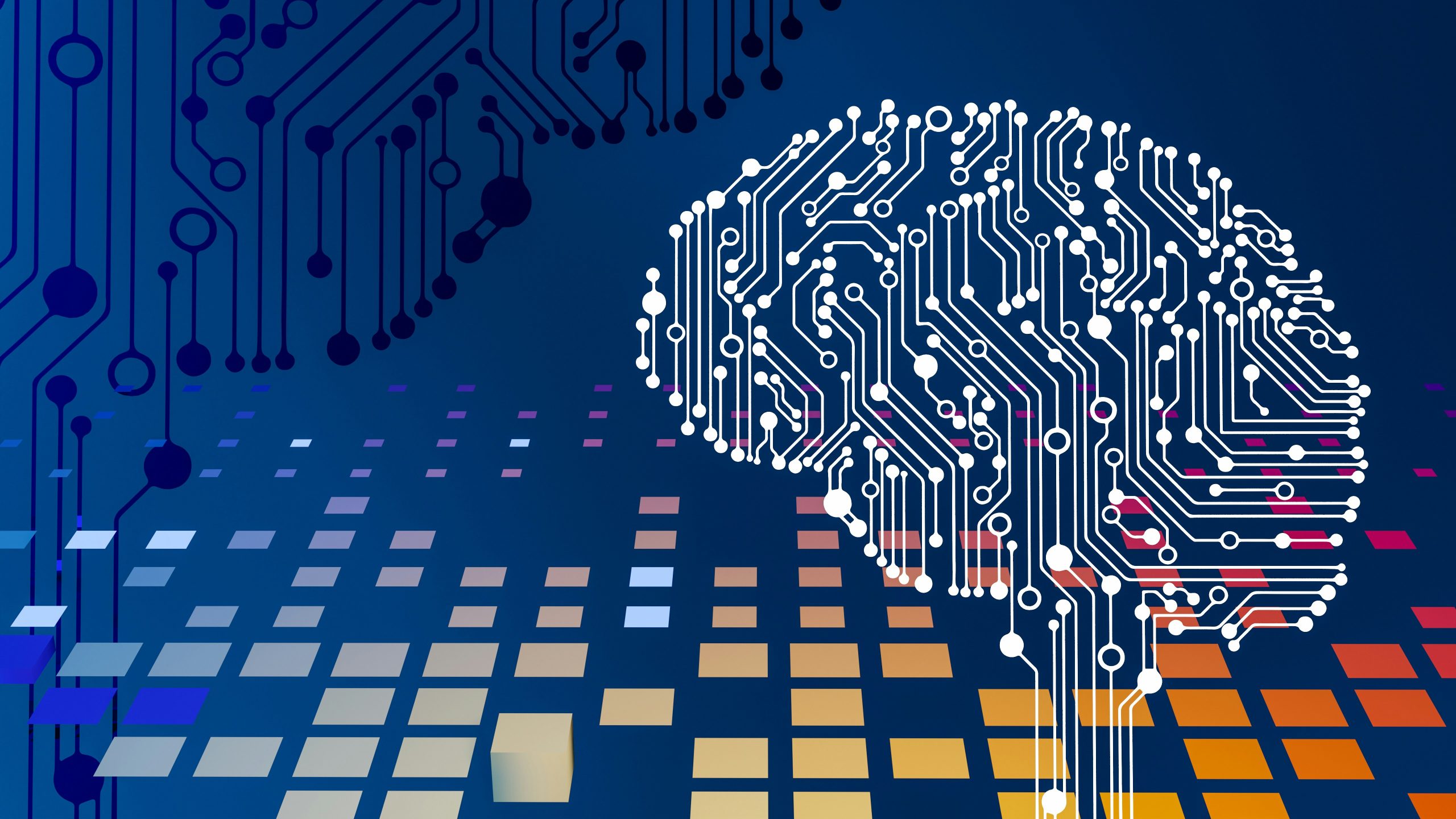
A recent McKinsey report found that 75% of large enterprises are investing in digital twins to scale their AI solutions. Combining digital twins with AI has the potential to enhance the effectiveness of large language models and enable new applications for AI in real-time monitoring, offering significant business and operational benefits.
What are digital twins?
Digital twins, originally developed to aid in the design of complex machinery have evolved significantly over the last two decades. They track and analyse live systems in real-time by processing device telemetry, detecting shifting conditions, and enhancing situational awareness for operational managers. Powered by in-memory computing, they enable fast, actionable alerts. Beyond real-time monitoring, digital twins also can simulate intricate systems like those for use in airlines and logistics, supporting strategic planning and operational decisions through predictive analytics.
Integrating digital twins with generative AI creates new opportunities for both technologies: The synergy can boost the prediction accuracy of generative AI, and can enhance the value of digital twins for system monitoring and development.
Proactively identifying anomalies with AI-powered digital twins
Continuous, real-time monitoring is a strategic necessity for organisations that manage complex live systems, like transportation networks, cybersecurity systems, and smart cities. Emerging problems must never be overlooked because delayed responses can cause small problems to become large ones.
Enhancing digital twins with generative AI reshapes how real-time monitoring interprets massive volumes of live data, enabling the reliable and immediate detection of anomalies that impact operations. Generative AI can continuously examine analytics results produced by digital twins to uncover emerging trends and mitigate disruptions before they escalate. While AI enhances situational awareness for managers, it can also pinpoint new opportunities for optimising operations and boosting efficiency.
At the same time, real-time data supplied by digital twins constrains the output of generative AI to avoid erratic results, like hallucinations. In a process called retrieval augmented generation, AI always uses the most up-to-date information about a live system to analyse behaviour and create recommendations.
Transforming data interaction with AI-driven visualisations
Unlocking insights from digital twin analytics should be intuitive, not technical. Generative AI is redefining how teams interact with massive datasets by enabling natural language-driven queries and visualisations. Instead of manually constructing intricate queries, users can simply describe their needs, and generative AI immediately visualises relevant charts and query results that provide new insights. This capability simplifies interactions and gives decision-makers the data they need. As organisations handle increasingly complex live systems, AI-powered intelligence allows them to efficiently sift through vast data pools, extract meaningful trends, and optimise operations with greater precision. It eliminates technical barriers, enabling faster, data-driven decisions that have a strategic impact.
Incorporating machine learning with automatic retraining
Digital twins can track numerous individual data streams and look for issues with the corresponding physical data sources. Working together, thousands or even millions of digital twins can monitor very large, complex systems. As messages flow in, each digital twin combines them with known information about a particular data source and analyses the data in a few milliseconds. It can incorporate a machine learning algorithm to assist in the analysis and find subtle issues that would be difficult to describe in hand-coded algorithms. After training with data from live operations, ML algorithms can identify anomalies and generate alerts for operational managers immediately.
Once deployed to analyse live telemetry, an ML algorithm will likely encounter new situations not covered by its initial training set. It may either fail to detect anomalies or generate false positives. Automatic retraining lets the algorithm learn as it gains experience so it can improve its performance and adapt to changing conditions. Digital twins can work together to detect invalid ML responses and build new training sets that feed automatic retraining. By incorporating automatic retraining, businesses gain a competitive edge with real-time monitoring that reliably delivers actionable insights as it learns over time.
Looking forward
Integrating digital twin technology with generative AI and ML can transform how industries monitor complex, live systems by empowering better real-time insights and enabling managers to make faster, more informed decisions. ScaleOut Software’s newly-released Digital Twins™ Version 4 adds generative AI using OpenAI’s large language model and automatic ML retraining to move real-time monitoring towards the goal of fully-autonomous operations.
(Image source: Unsplash)